September 2022
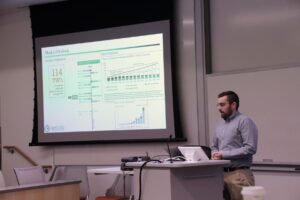
My first contribution to teaching was back in 2008 as an assistant to Prof. Korres and on the graduate-level subject on machine learning (ML) applications within power system control centers. The subject was more of a review on research ideas and publications exploring if and how ML could be useful in real-time operation of electrical grids, as also the planning of their infrastructure. As a junior PhD student under Prof. Hatziargyriou at that time I was just then dipping my toes in the vast sea of research on artificial intelligence (AI) in power systems. Helping teach that course offered some valuable insights.
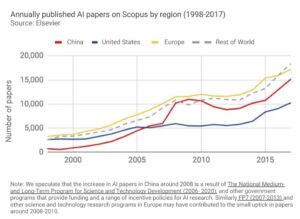
In those years, ML and AI were very appealing in the mainstream engineering education and research (buildings, components, power systems, circuits, etc), but were themselves going through some introspection within the computer science community. There are several articles pointing out to the relative plateauing of R&D on AI & ML in the later 2005-10 period, followed by exponential growth afterwards. Nevertheless, more traditional engineering fields were warming up to their “digitalization” and reinventing themselves as smart: smart grids, smart buildings, smart materials and so on. Even though this latter push was strong within research circles and several professional initiatives started popping up, in practice, AI & ML applications were taking baby-steps. The tide changed after bold start-ups adopted their value en masse in the mid 2010s.
Looking back to the period of 2005-10 through the lens of the technology shifts of the later years, made me realize something ‘big’ about higher education teaching. We do not teach to only educate or provide necessary skill-sets; we teach to seed a vision. And I am very cautious with my words here. I do not proclaim that university teaching must be ‘persistently’ forward-thinking. I do not imply that the fundamentals should be in any way glossed over. I do not believe that there is such a thing as either redundant or rudimentary knowledge. What I am saying though is that higher-ed teachers have a unique challenge: we must read the tea leaves or crystal balls of our “craft” and prepare students of what is to come, even though no one else might be seeing this yet.
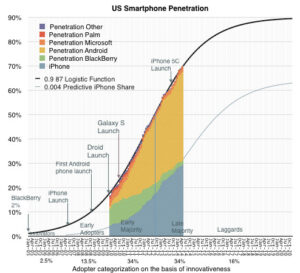
Think about the example of the course I mentioned earlier and the ‘smartening up’ of many economy sectors. The actual computer science field was kind of taking a breather on AI and ML at that time, yet all other engineering fields were bracing confidently about a tech boom relying exactly on AI and ML! If we seek to dissect this paradox a bit, we will see that there were – actually – no tea leaves or crystal balls necessary. Computers and mobile devices were becoming widely available and used a ton by consumers, practically giving educators the low-hanging fruit of inspiration about advanced computing tools.
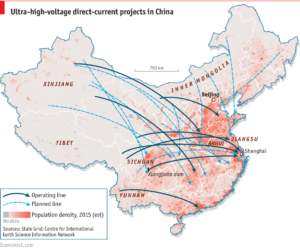
Fast forward to 2018, when I developed, proposed to CMU and started teaching a course, then titled, “Optimization Modeling in Power Systems”. I was reading my “crystal ball” and realizing that the ever present discussion about the failing and ageing grids (first pointed out in the 1990s) was actually spilling dangerously into reality. Add another 3-4 years to that and here we are in 2021 and 2022 witnessing the passing of multi-billion dollar bills that will expand the US electrical grid, several start-ups monetize efficiency for end-customers globally, while China has already taken strides ahead in its high-voltage transmission system backbone. Optimizing all electrical grid operations and planning, which used to be the expertise of engineers with PhDs, is now expected by the MSc graduates of energy engineering programs. My personal story here is that in 2018 and the next couple of years, the “Optimization Modeling in Power Systems” course was attended by barely 5 students – mostly in their PhDs; now I teach a roster of 16 students – more than half of whom are MSc students.
What I mean to point out with the above story is that power and energy engineering education is not straight-forward, since it links to multiple other subjects and fields. I am sad to attest to statements of scholars I admire saying that “power engineering is not science”. And yet here we are, following a “hunker down pandemic” (which should have reduced energy demand) and a 6-month localized war (which should have not hurt international energy security), which are crippling multiple economies by threatening electricity markets everywhere. Even more ironically, most of these electricity markets could have already had energy independence, had they built out their grids and/or made them much more efficient. Put simply, energy and power educators have to look deep and wide in their research and expertise, and aspire to new subjects and angles (decentralized? autonomous? self-sufficient? resilient?). The energy and power work force is crumbling, several places around the world are still not electrified consistently (let alone have energy security), and energy dependencies are heavy and stretch the globe.
I conclude this blog with a final thought. The energy sector comprises entities and processes that are complex, large and slow to adapt, while the challenges they are faced with and the tools to address them are fast and with much impact. This means that the teaching aspirations of power and energy engineering faculty must be also realistic and delivered with confidence and persistence. As instructors in this field, we must develop the necessary intuition and retain adequate humility in keeping our ear to the ground for the right signs of change. And this last thing is not easy; personally, I have acquired these “skills” with years of practicing engineering and humbling disappointment across several projects I have worked on. Others before and around me are trying through broad involvement in committees, initiatives and working groups. Whatever the way, educating the next generations of power and energy engineers is an urgent duty hanging already over our heads, especially, if we wish to be honest to our vision for the clean energy transition.